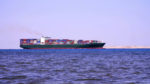
The supply chain as we know it is on the precipice of tipping from decades of steadily accelerating “push” dynamics to a new “push-and-pull” model.
Four main factors are contributing to this global, industry-wide change:
Today’s increasingly savvy shoppers. Customers live in the digital world and demand a seamless experience. If not, they’ll go elsewhere. This means that the supply chain — which is optimized for “pushing” inventory to customers — needs to add optimization for what customers wants to “pull” to themselves.
The current geopolitical climate. Whether it’s the nationalistic tendencies on display globally, tariff disputes between the U.S. and China, Brexit, or the global focus on sustainability issues, supply chains are more than ever exposed to uncertainty and risk.
Uneven advances in Industry 4.0 and digital supply chain. Factories, supply chains, and stores are becoming more connected, enabling different systems to share information and shrink lead times — but only for companies that are able to act. Production is shifting closer to customers, disrupting long-held patterns of regional trade.
Existing supply-chain technology is at the end of its lifecycle. Legacy software solutions, built to solve a specific, isolated problem such as forecasting or factory planning, are no longer fit for the purpose. To put it simply, this software just can’t keep up.
Enter artificial intelligence for the supply chain. Be it through predictive maintenance in the factory, self-driving trucks in the logistics chain, or automation in the store, AI solutions are emerging to improve efficiency and lower operating costs for supply-chain players. Yet there's a disconnect, as in most industries, around how to fully recognize value from AI.
The first step is to create a roadmap: a portfolio of vetted AI opportunities prioritized to achieve strategic business goals over the short and long term. Learning by experiment remains critical for adopting AI, but having an AI roadmap is a necessary strategy. It allows those dealing with improving supply-chain logistics to plan and choose the best tactics for intelligent AI adoption.
How do you develop an AI roadmap to capture the momentum of the changing supply chain in your business? First, you need to scope the work at the level of a business line or functional area — not the whole business, nor a single process or task. In a factory setting, for example, areas such as inventory or quality management are good places to start because they represent complete systems of processes, data, roles, and goals.
After choosing your focus, you’ll need to educate your executives on what a good AI opportunity looks like, then work across your team to tap into possibilities for matching AI capabilities to opportunities.
Good AI opportunities are both practical and valuable. They address which prediction or decision will be made by AI, using what data, and how that output will be applied to create value. In factory quality management, for example, AI systems could use production line data to predict defects, prescribe maintenance, or augment analysts in answering questions.
Discovery is as much about finding these options as it is about forming a hypothesis regarding which ones are worth pursuing. After discovery, your team should have a set of case studies that now need to be validated.
When estimating the impact of an AI use case, look at more than just incremental improvements to accuracy or efficiency. Analyze the value created above and beyond the status quo.
For example, an AI use case could be built around helping an accounts payable clerk who regularly reads reports and processes invoices. With an AI capability such as document intelligence, simple invoices can be handled by straight-through processing. The clerk can then handle remaining invoices more quickly, assisted by features like automatic document summary and information extraction, such as for discrepancy identification. An initial metric for this benefit could simply be invoices processed or time saved, but there’s a bigger picture. If an AP clerk gains an extra hour per day, they could spend it on root-cause analysis and correction of order issues, or managing their workload to take better advantage of supplier discounts.
The analysis phase is done when each case has been defined to the point that executive decisionmakers have enough information to make tough conclusions about prioritizing cases in a vision and plan.
Finally, with well-defined use cases in hand, your team is ready to sequence AI investments for maximum impact by balancing three goals:
- Setting up immediate next steps with valuable, actionable projects,
- Setting up bigger wins with a deliberate plan for building and scaling capabilities over time, and
- Unlocking support and budget by aligning executives around a common vision.
The first two goals are about balancing short- and long-term value. The third is about unblocking progress by securing buy-in from executive leadership about the realistic costs and benefits of AI investments in the supply chain.
As the supply chain tips to a new responsive, push-and-pull normal, it will be increasingly vital for decisionmakers to act quickly to seize the momentum. However, the intricacies of supply-chain management dictate that rebalancing supply chain equilibrium is not a one-and-done activity. Taking the time to discover, analyze, and prioritize your AI investments will make all the difference between getting ahead — or wasting your time.
Karthik Ramakrishnan is VP, Head of Advisory and Enablement, and Ben Humphries is Head of Global PreSales, at Element AI.